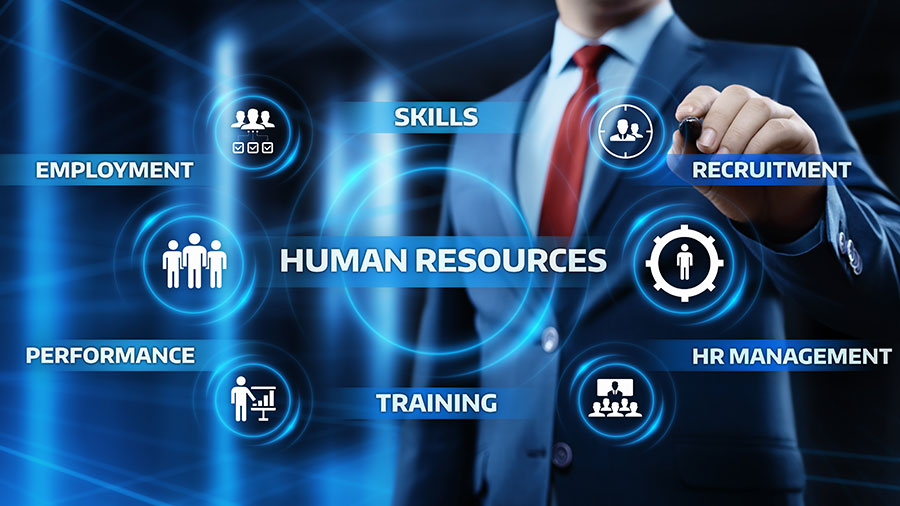
In the ever-evolving landscape of human resources management (HRM), technology has emerged as a powerful ally, reshaping traditional practices and enhancing decision-making processes. Among the forefront technologies driving this transformation are Artificial Intelligence (AI), Data Science, and Analytics. This article explores their pivotal role in HRM Analytics, showcasing how organizations are leveraging these technologies to streamline operations, optimize workforce management, and foster a data-driven HR culture.
I. Talent Acquisition and Recruitment:
AI and Data Science have significantly impacted the talent acquisition process. Intelligent algorithms can analyze resumes, screening candidates based on predefined criteria, and even predict the likelihood of a candidate’s success in a particular role. Tools like HireVue use AI to assess candidates’ facial expressions, voice tone, and language, providing deeper insights into their suitability for a position. This not only expedites the hiring process but also enhances the quality of candidate selection.
Here are some ways in which AI technologies are contributing to the HRM domain in Talent Acquisition:
- Resume Screening and Candidate Matching:
AI-powered tools can analyze vast amounts of resumes quickly and efficiently. Machine learning algorithms can learn from historical hiring data and identify patterns associated with successful hires. This enables HR professionals to screen resumes based on specific criteria, such as skills, experience, and qualifications, automating the initial stages of the recruitment process.
- Predictive Analytics for Candidate Success:
Data Science is employed to develop predictive analytics models that assess the likelihood of a candidate’s success in a particular role. By analyzing historical performance data, these models can predict which candidates are most likely to excel in a given position. This not only speeds up the hiring process but also improves the chances of making successful hires.
- Chatbots for Initial Candidate Interaction:
AI-driven chatbots are being used for initial candidate interactions. These chatbots can engage with candidates, answer frequently asked questions, and collect basic information. By automating the initial stages of communication, HR professionals can focus on more complex tasks, while candidates receive a prompt and consistent experience.
- Video Interviewing and Analysis:
Video interviewing platforms utilize AI to analyze candidates’ facial expressions, voice tone, and language during interviews. This technology, often referred to as sentiment analysis, provides insights into a candidate’s soft skills, emotional intelligence, and cultural fit. Tools like HireVue use AI to evaluate non-verbal cues, helping recruiters make more informed decisions about a candidate’s suitability for a role.
- Behavioral Analysis in Social Media:
AI and Data Science are employed to analyze candidates’ online presence, including social media profiles. This allows recruiters to gain insights into a candidate’s behavior, interests, and overall suitability for the organization’s culture. While respecting privacy and ethical considerations, this information can contribute to a more comprehensive understanding of potential candidates.
II. Employee Engagement and Retention:
Maintaining a high level of employee engagement and reducing turnover is a critical concern for HR professionals. AI-driven analytics tools analyze employee feedback, sentiment, and behavior, helping organizations identify factors contributing to job satisfaction or dissatisfaction. IBM Watson, for instance, provides AI-driven insights into employee sentiments by analyzing surveys, reviews, and communication channels. By addressing key concerns, HR departments can implement targeted strategies to improve overall employee satisfaction and retention.
Here are some ways in which AI and Data Science contribute to employee engagement and retention:
- Sentiment Analysis:
AI-powered sentiment analysis tools examine employee feedback, surveys, and communication channels to gauge overall sentiment within the organization. By analyzing language, tone, and context, these tools can identify areas of concern or satisfaction among employees. This allows HR teams to address potential issues proactively and implement strategies to improve overall morale.
- Predictive Analytics for Turnover Risk:
Data Science models can predict turnover risk by analyzing various factors such as job satisfaction, work-life balance, and historical turnover patterns. By identifying employees at higher risk of leaving, HR departments can implement targeted retention strategies, such as personalized career development plans, mentorship programs, or adjustments to work arrangements.
- Personalized Employee Feedback:
AI-driven surveys and feedback tools can provide personalized insights to employees based on their responses. These tools analyze individual feedback and offer tailored suggestions for improvement or areas of recognition. By addressing specific concerns and acknowledging achievements, organizations can foster a culture of continuous improvement and positive reinforcement.
- Employee Recognition and Rewards:
AI helps in identifying and recognizing employee achievements through data analytics. By analyzing performance metrics, project outcomes, and peer feedback, AI can recommend personalized recognition and reward programs. This not only boosts employee morale but also reinforces a culture of appreciation, contributing to higher engagement and retention rates.
Predictive Modeling for Employee Development:
Data Science models can predict the career path and development trajectory of individual employees based on their skills, performance, and training history. This allows HR departments to proactively plan career development opportunities, ensuring that employees are engaged and motivated by a clear growth path within the organization.
III. Workforce Planning and Optimization:
Data Science plays a pivotal role in workforce planning, ensuring that organizations have the right talent in the right place at the right time. Predictive analytics models can forecast future workforce needs based on historical data, industry trends, and organizational goals. This enables HR managers to proactively address skills gaps, plan training programs, and align the workforce with the company’s strategic objectives. Workday’s Adaptive Insights is an example of a tool that combines AI and analytics for robust workforce planning.
Here are several ways in which AI and Data Science contribute to workforce planning and optimization:
- Predictive Analytics for Skill Gaps:
AI-driven predictive analytics models analyze historical data, current skill sets, and emerging trends to identify potential skill gaps within the workforce. By forecasting future skills requirements, HR professionals can proactively plan training programs, recruitment strategies, and succession planning to ensure the organization is equipped with the right talent.
- Talent Acquisition Forecasting:
AI and Data Science tools predict future workforce needs by analyzing factors such as business growth, industry trends, and historical hiring patterns. This enables HR departments to develop recruitment strategies well in advance, ensuring that the organization has the right talent at the right time.
Succession Planning:
AI and Data Science models assess employee performance, skills, and career trajectories to identify potential candidates for key roles within the organization. Succession planning tools use predictive analytics to ensure that there are qualified individuals ready to step into critical positions when needed, reducing disruptions and ensuring continuity.
- Workforce Optimization Models:
AI-driven optimization models analyze various workforce scenarios to find the most efficient and cost-effective staffing configurations. This includes evaluating factors such as part-time vs. full-time employees, outsourcing options, and flexible work arrangements. These models help HR teams make informed decisions to optimize workforce efficiency.
Demand Forecasting for Project Staffing:
AI and Data Science analyze project-related data to forecast staffing needs for specific initiatives or projects. By understanding the skills required and the duration of projects, HR professionals can allocate resources efficiently, ensuring that the organization has the right talent to deliver successful outcomes.
- Adaptive Workforce Planning:
AI enables HR departments to adapt their workforce planning strategies dynamically in response to changes in the business environment. Whether it’s a sudden market shift, technological advancements, or unforeseen events, adaptive workforce planning ensures that organizations can quickly realign their talent strategies to stay agile and competitive.
IV. Performance Management:
Traditional performance evaluations are often criticized for being subjective and biased. AI-driven performance management tools eliminate such biases by objectively analyzing an employee’s performance data. These tools provide real-time feedback, track key performance indicators, and help employees set personalized goals. Adobe’s AI-powered tool, “Sensei,” assesses employee performance and provides actionable insights for continuous improvement, fostering a culture of ongoing development.
Here are several ways in which AI and Data Science contribute to performance management:
- Objective Performance Metrics:
AI enables the creation of objective performance metrics by analyzing quantitative data such as project completion rates, sales figures, and key performance indicators (KPIs). This helps HR professionals and managers evaluate employee performance based on tangible and measurable outcomes, reducing the impact of subjective biases.
- Continuous Performance Monitoring:
AI-powered tools provide real-time monitoring of employee performance. This continuous tracking allows HR teams to identify trends, patterns, and potential issues as they emerge, enabling proactive interventions. It also facilitates ongoing feedback, fostering a culture of continuous improvement.
- 360-Degree Feedback Analysis:
Data Science is employed to analyze 360-degree feedback from various sources, including peers, subordinates, and supervisors. AI algorithms identify recurring themes and patterns in feedback, providing a comprehensive view of an employee’s strengths and areas for improvement. This holistic approach ensures a well-rounded evaluation of an individual’s performance.
- Predictive Analytics for Performance Trends:
Predictive analytics models use historical performance data to identify trends and patterns, allowing HR professionals to predict future performance outcomes. This helps in proactively addressing potential performance issues, aligning resources effectively, and optimizing talent development initiatives.
Real-time Feedback and Recognition:
AI facilitates real-time feedback mechanisms by automatically analyzing and flagging performance achievements or areas for improvement. This allows managers to recognize and reward positive contributions promptly and address performance issues in a timely manner, contributing to employee motivation and satisfaction.
V. Learning and Development:
AI enhances learning and development initiatives by personalizing training programs based on individual employee needs. Analyzing employee performance data, preferences, and learning styles, AI algorithms recommend customized learning paths. Cornerstone OnDemand, an AI-driven learning platform, employs predictive analytics to identify skill gaps and suggest relevant training courses, empowering employees to upskill and stay relevant in their roles.
Here are several ways in which AI and Data Science contribute to learning and development:
- Personalized Learning Paths:
AI analyzes employee skills, performance data, and learning preferences to recommend personalized learning paths. This ensures that training programs are tailored to individual needs, maximizing the relevance and effectiveness of the content. Adaptive learning platforms use real-time data to adjust the pace and difficulty of materials based on an employee’s progress.
- Predictive Analytics for Skill Gaps:
Data Science models predict skill gaps within the workforce by analyzing current skill sets, performance data, and industry trends. This information guides HR professionals in developing targeted training programs to address specific skill deficiencies, ensuring that the workforce remains aligned with organizational goals.
- Content Recommendation Engines:
AI-powered recommendation engines analyze employee profiles, learning history, and performance data to suggest relevant training materials and courses. By understanding individual learning preferences and skill gaps, these engines enhance the discovery of content that is most beneficial for each employee.
- Assessment and Feedback Automation:
AI automates the assessment and feedback processes in training programs. This includes the use of machine learning algorithms to evaluate quiz and test results, providing instant feedback to learners. Automated assessments not only save time but also contribute to a continuous learning environment.
- Generative AI for Learning Interaction:
Generative AI algorithms and NLP enhance the interaction between employees and learning platforms through applications like Chat GPT and Chatbots. AI applications powered by Generative AI can answer questions, provide clarification, provide a detail and specific information, summarise books, help in finding out where a specific concept was discussed in a book, etc. This fosters a more engaging and user-friendly learning experience.
- Gamification for Enhanced Engagement:
AI is employed in gamification elements within learning platforms to increase engagement. By incorporating game-like features such as badges, leaderboards, and interactive simulations, AI-driven gamification encourages employees to actively participate in training programs, making learning more enjoyable and effective.
- Employee Skill Profiling:
Data Science creates skill profiles for each employee by analyzing their performance data and completed training modules. These profiles assist HR professionals in identifying strengths, weaknesses, and potential areas for improvement. Skill profiling enables targeted development plans and helps in succession planning.
VI. Employee Well-being:
In the modern workplace, employee well-being is a crucial aspect of HRM. AI and data analytics tools monitor various well-being indicators such as stress levels, workload, and work-life balance. Microsoft’s Workplace Analytics, for instance, provides insights into employee collaboration patterns, helping organizations identify potential burnout risks. By proactively addressing these concerns, HR can contribute to a healthier and more productive work environment.
Here are several ways in which AI and Data Science contribute to promoting well-being in the workplace:
- Stress and Burnout Prediction:
AI powered applications can analyzes various data sources, including communication patterns, workload, and project deadlines, to predict stress and burnout levels among employees. By identifying early signs of potential issues, HR teams can intervene with targeted support, such as workload adjustments, flexible schedules, or access to mental health resources.
- Well-being Surveys and Sentiment Analysis:
AI-driven surveys and sentiment analysis tools gather feedback from employees about their well-being. By analyzing responses, HR professionals can identify trends and areas of concern. This data-driven approach enables organizations to tailor well-being initiatives based on real-time insights from employees.
- Personalized Well-being Programs:
AI analyzes individual health and well-being data to recommend personalized well-being programs for employees. These programs may include fitness challenges, mindfulness sessions, or access to mental health resources. Personalization ensures that employees receive support tailored to their unique needs and preferences.
- Proactive Mental Health Support:
AI-driven tools monitor communication patterns and language use to detect signs of mental health issues. By identifying early indicators, HR professionals can offer proactive support, such as access to counseling services or mental health resources, contributing to a workplace culture that prioritizes mental well-being.
Conclusion:
The integration of Artificial Intelligence (AI), Data Science, and Analytics has ushered in a transformative era in Human Resources Management (HRM), revolutionizing traditional practices. These technologies have become indispensable tools for HR professionals, empowering them to make informed decisions, optimize workforce management, and foster a data-driven organizational culture. In talent acquisition, AI algorithms streamline the recruitment process, screening resumes, and predicting candidate success. Data Science plays a pivotal role in workforce planning, aligning talent with organizational goals through predictive analytics. AI-driven performance management tools eliminate bias, providing objective evaluations and real-time feedback. Learning and development initiatives benefit from personalized training programs recommended by AI algorithms, ensuring employees acquire skills tailored to their needs. Additionally, AI and analytics contribute to employee well-being by monitoring stress levels and collaboration patterns. This synergy of technologies is not merely a competitive advantage but a necessity for HRM, allowing organizations to adapt and excel in the dynamic landscape of talent management.
Jayant S. Ramteke
Founder and Managing Director, Meritorium Knowledge Academy
FRM GARP, B Tech IIT B, PGDM IIM C, EDP Cornell